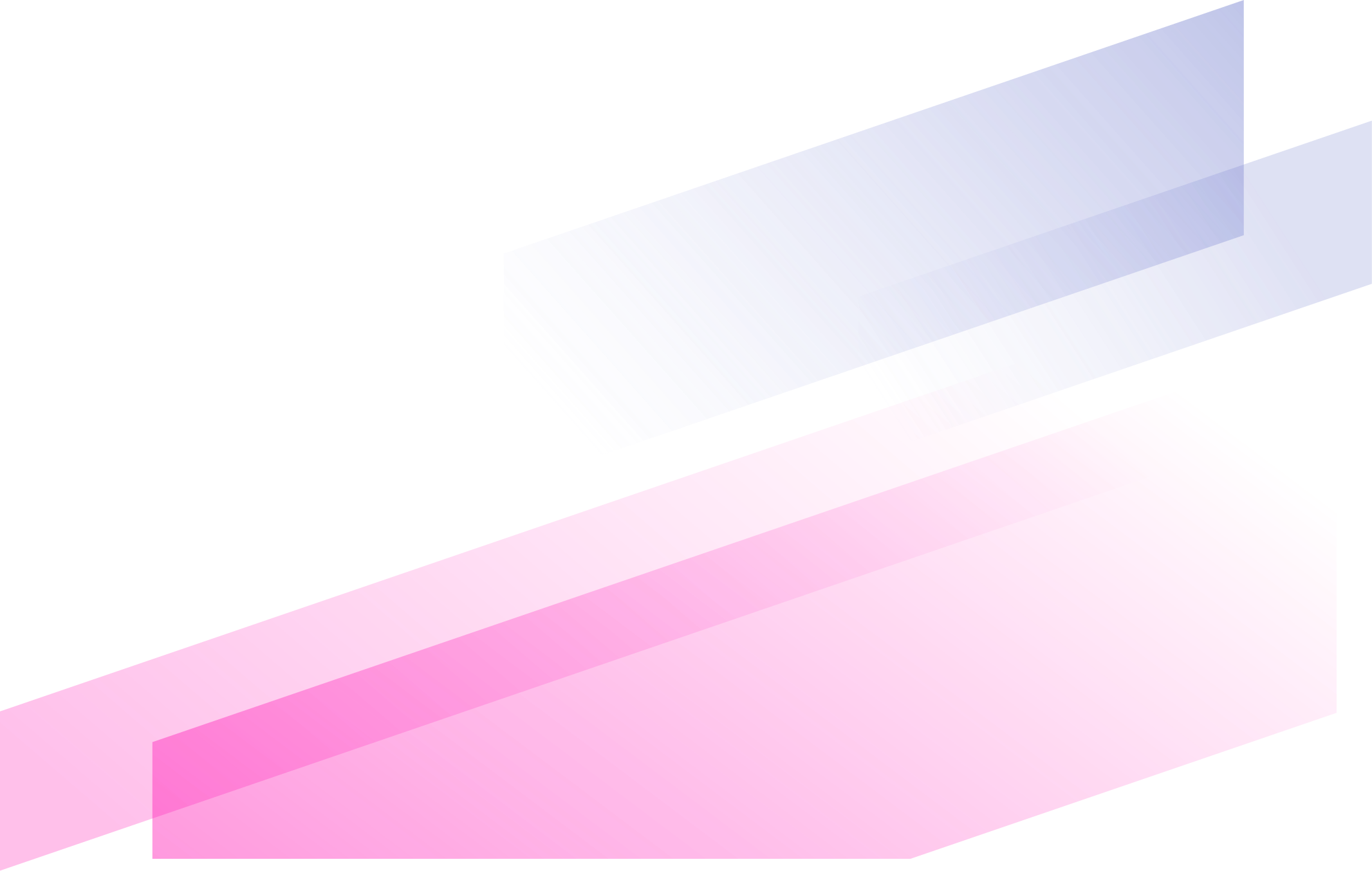
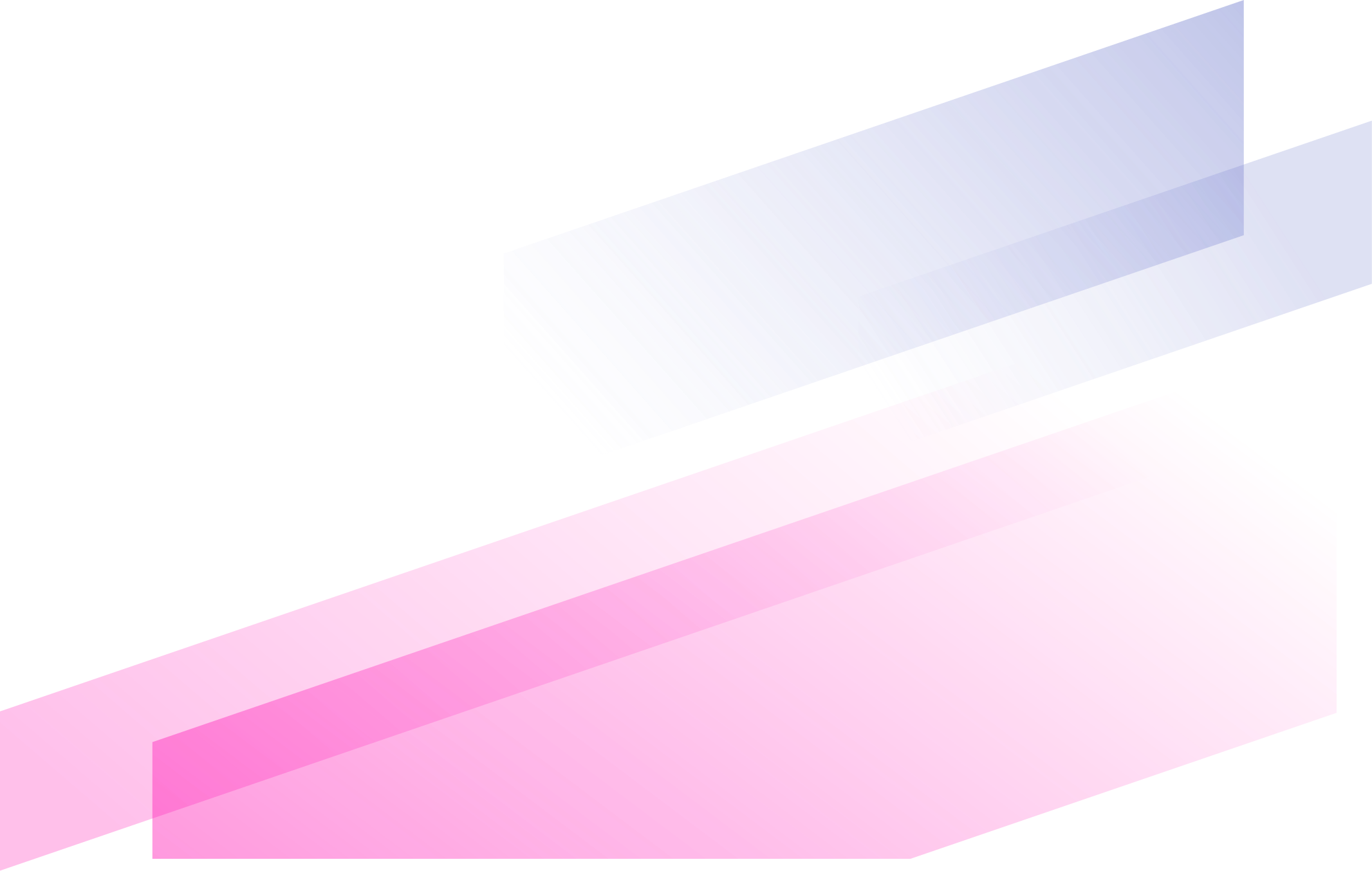
3D-ALMOND-QSAR Models to Predict the Antidepressant Effect of Some Natural Compounds.
Investigation of structural analogs of hydroxychloroquine for SARS-CoV-2 main protease (Mpro): A computational drug discovery study.
Accelerating antibiotic discovery through artificial intelligence.
DTI-HeNE: a novel method for drug-target interaction prediction based on heterogeneous network embedding.
Prediction of Drug-Target Interactions by Combining Dual-Tree Complex Wavelet Transform with Ensemble Learning Method.
FORUM: Building a Knowledge Graph from public databases and scientific literature to extract associations between chemicals and diseases.
ExonSkipAD provides the functional genomic landscape of exon skipping events in Alzheimer's disease.
Deciphering osteoarthritis genetics across 826,690 individuals from 9 populations.
Human melanocyte development and melanoma dedifferentiation at single-cell resolution.
A machine learning framework for predicting drug-drug interactions.