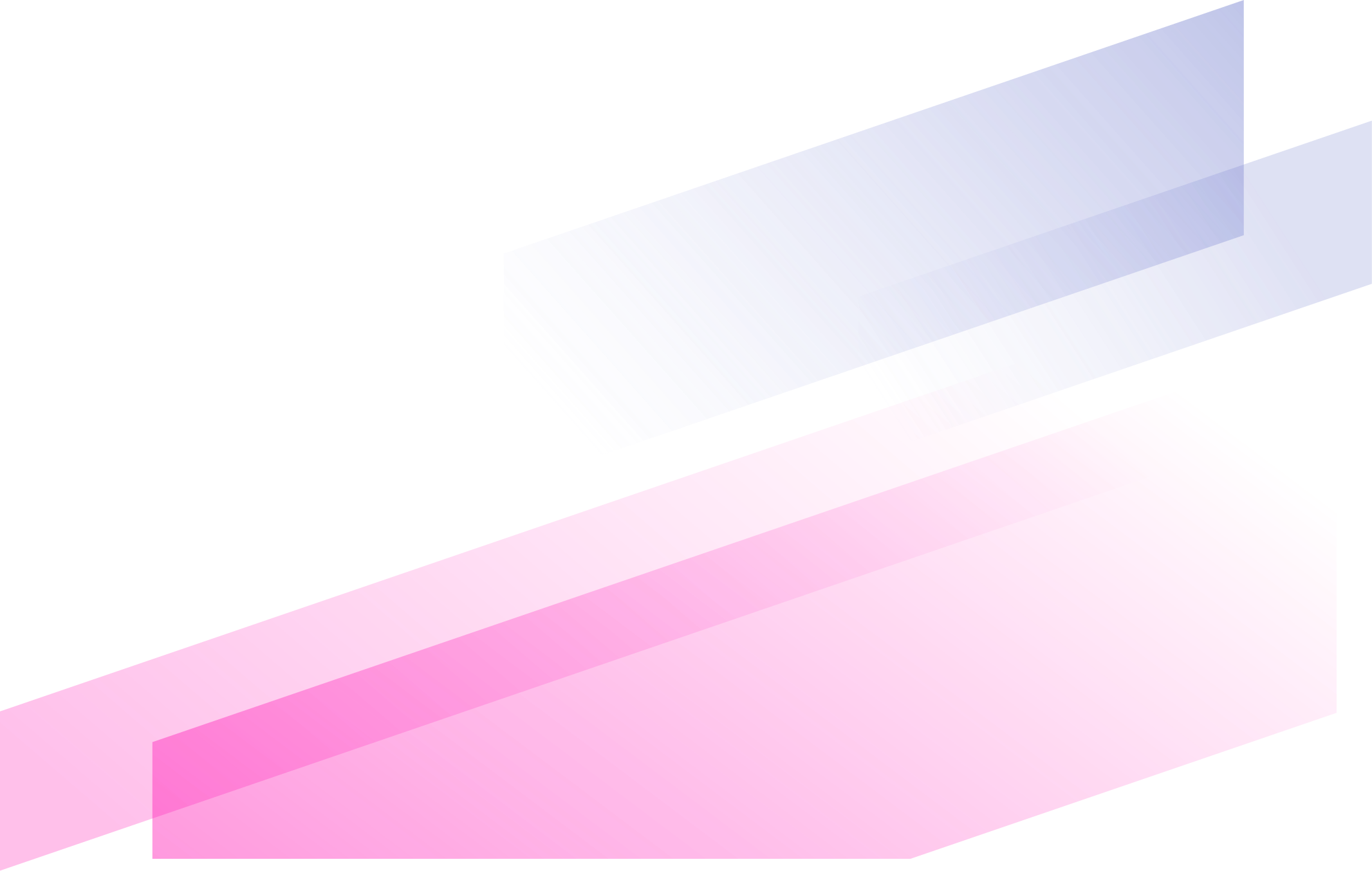
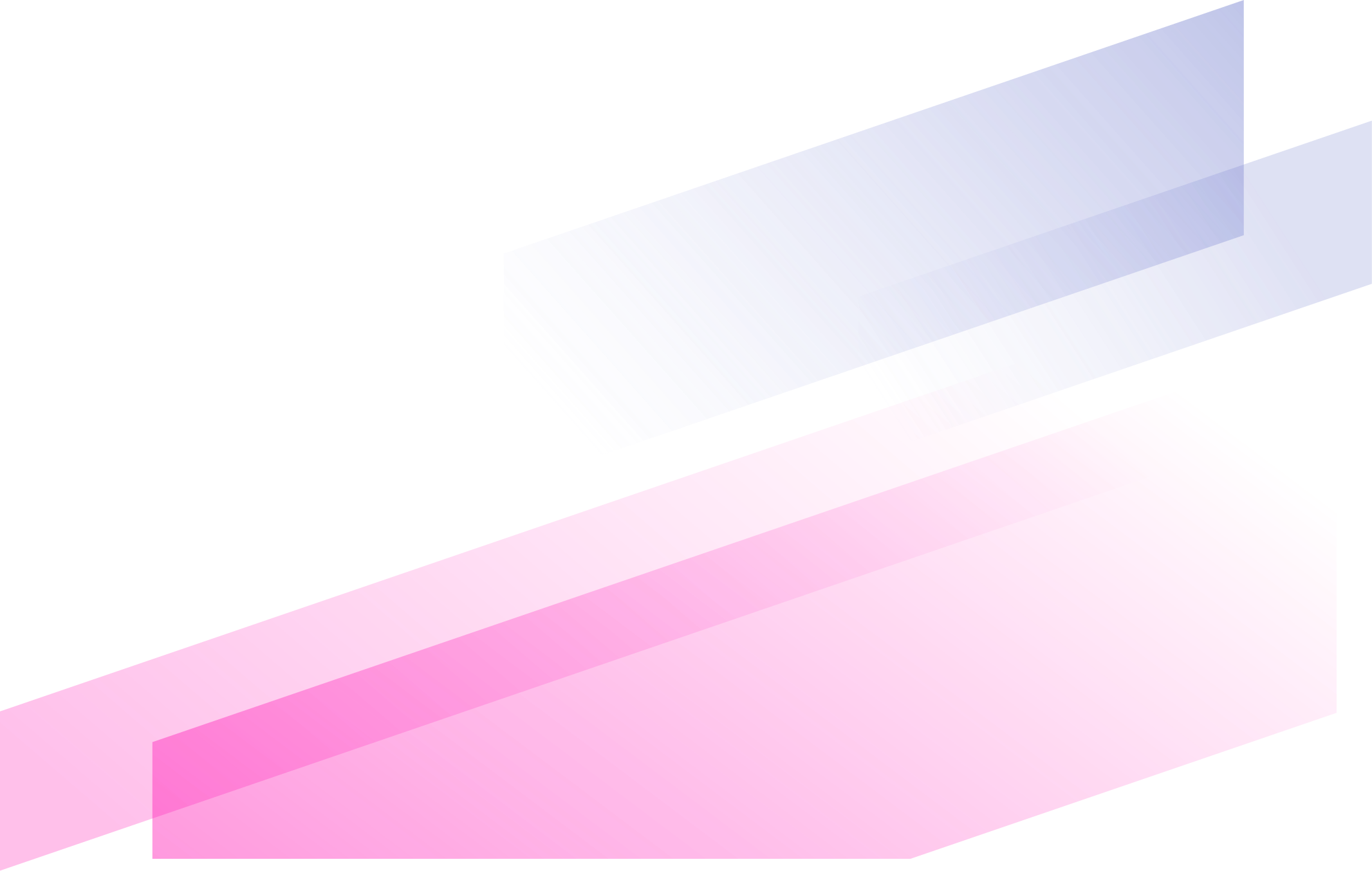
Integrated Bioinformatics Analysis of DNA Methylation Biomarkers in Thyroid Cancer Based on TCGA Database.
Functional comparison of exome capture-based methods for transcriptomic profiling of formalin-fixed paraffin-embedded tumors.
Repurposing FDA Drug Compounds against Breast Cancer by Targeting EGFR/HER2.
Multimorbidity prediction using link prediction.
Protein Integrated Network Analysis to Reveal Potential Drug Targets Against Extended Drug-Resistant Mycobacterium tuberculosis XDR1219.
GraphDTI: A robust deep learning predictor of drug-target interactions from multiple heterogeneous data.
Rare variant contribution to human disease in 281,104 UK Biobank exomes.
Comparative transcriptome analyses reveal genes associated with SARS-CoV-2 infection of human lung epithelial cells.
The Antimalarial Mefloquine Shows Activity against Mycobacterium abscessus, Inhibiting Mycolic Acid Metabolism.
NetControl4BioMed: A web-based platform for controllability analysis of protein-protein interaction networks.